- 2002 22
- 2003 86
- 2004 72
- 2005 102
- 2006 74
- 2007 42
- 2008 46
- 2009 48
- 2010 26
- 2011 55
- 2012 141
- 2013 126
- 2014 241
- 2015 320
- 2016 291
- 2017 338
- 2018 463
- 2019 450
- 2020 391
- 2021 271
- 2022 266
- 2023 263
- 2024 120
- 2025 1
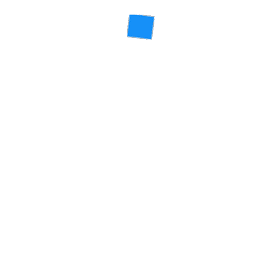
Estatística Anual da Produção Científica Docente do Inatel
Soma geral: 4255
Dados obtidos através CVLattes dos Docentes em 02/2025.